In the ever-evolving landscape of technology, edge computing has emerged as a transformative paradigm, pushing data processing closer to the source rather than relying solely on centralized cloud systems. From smart homes to industrial IoT, edge computing optimizes efficiency, reduces latency, and enhances real-time decision-making. Yet, nowhere is its potential more vividly realized than in Full Self-Driving (FSD) systems—autonomous vehicles that represent the zenith of edge computing’s capabilities.
What is Edge Computing?
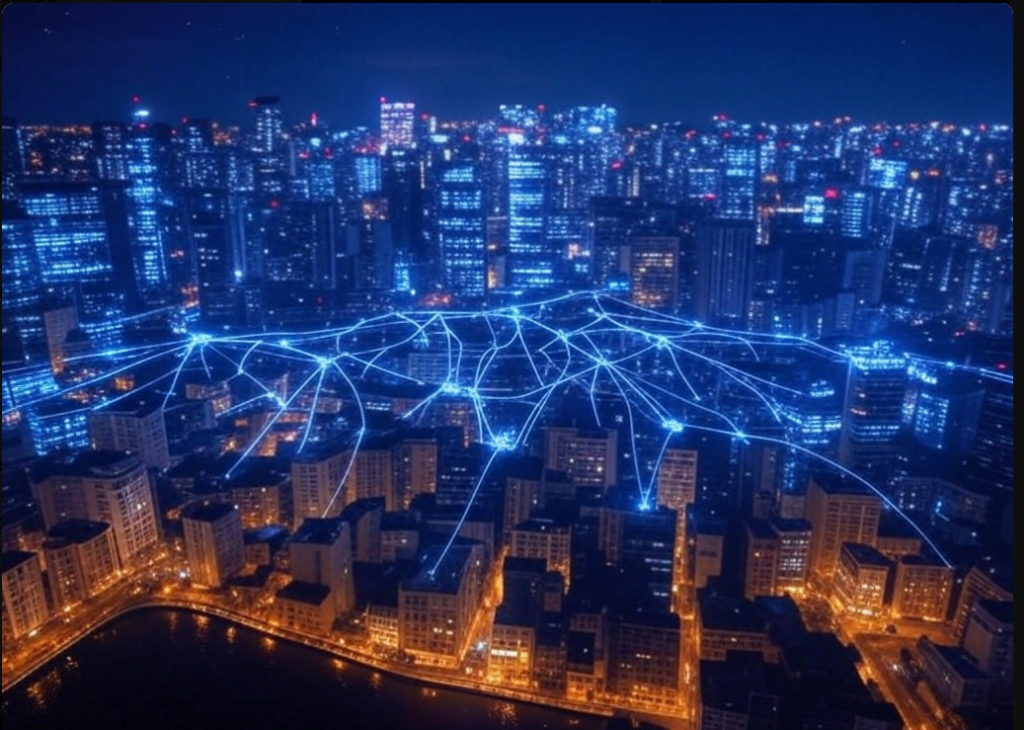
At its core, edge computing involves processing data at or near the location where it is generated, rather than transmitting it to a distant server or cloud for analysis. This approach minimizes delays, reduces bandwidth usage, and ensures functionality even in environments with limited connectivity. In a world increasingly defined by real-time demands, edge computing is the backbone of systems requiring split-second responsiveness.
Now, imagine a scenario where edge computing must handle vast streams of data, make life-or-death decisions, and adapt to unpredictable conditions—all while moving at 70 miles per hour. This is the reality of Full Self-Driving technology, as exemplified by pioneers like Tesla, Waymo, and others. FSD isn’t just an application of edge computing; it’s the ultimate test of its limits.
The Edge Computing Demands of FSD
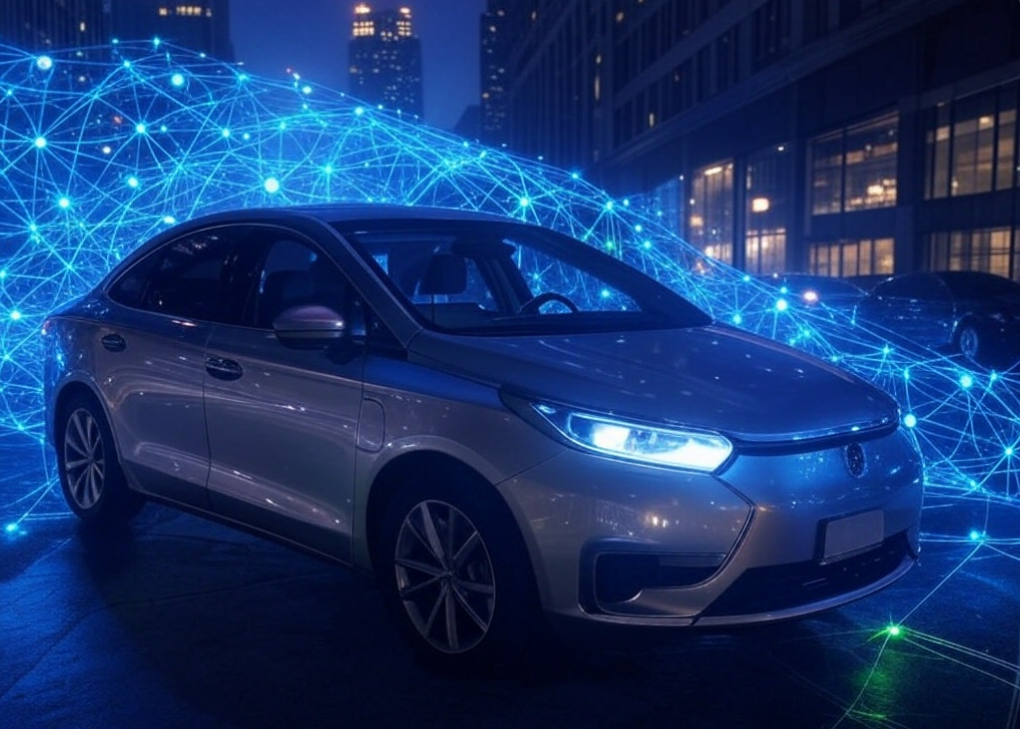
An autonomous vehicle is a rolling supercomputer, bristling with sensors—cameras, radar, LIDAR, ultrasonic detectors—that generate terabytes of data daily. This data includes everything from lane markings and traffic signals to the erratic movements of pedestrians and the sudden appearance of a deer on a rural road. Processing this information in the cloud would introduce unacceptable latency; even a half-second delay could spell disaster at highway speeds. Instead, FSD systems rely on onboard edge computing to interpret, analyze, and act on this data instantaneously.
Tesla’s approach to FSD, for instance, leverages a custom-built Hardware 3 (and now Hardware 4) chip designed specifically for neural network computations. This hardware, paired with sophisticated software, processes sensor inputs in real time to predict trajectories, recognize objects, and execute driving maneuvers. The car itself is the “edge”—a self-contained unit making autonomous decisions without constant reliance on external servers. While over-the-air updates and cloud-based training refine the system, the moment-to-moment operation happens entirely on the vehicle.
Why FSD is the Ultimate Edge Computing Challenge
- Real-Time Processing at Scale
Unlike a smart thermostat adjusting temperature or a security camera recognizing a face, FSD must process a multidimensional, dynamic environment. It integrates inputs from dozens of sensors, fuses them into a coherent model of the world, and updates that model thousands of times per second. This demands computational power and efficiency far beyond typical edge applications. - Safety-Critical Decision-Making
Errors in most edge systems—like a delayed smart speaker response—are inconvenient but rarely catastrophic. In contrast, FSD errors can have immediate, irreversible consequences. Edge computing in autonomous vehicles must achieve near-perfect reliability, balancing speed with precision in ways other applications rarely demand. - Adaptability to Edge Cases
The road is a chaotic tapestry of edge cases—weird weather, erratic drivers, construction zones, and unpredictable obstacles. FSD systems must generalize from training data while adapting to scenarios they’ve never encountered, all without phoning home for help. This requires advanced machine learning models optimized to run on local hardware—a hallmark of edge computing’s evolution. - Resource Constraints
Vehicles have limited power, space, and cooling capacity compared to cloud data centers. FSD pushes edge computing to maximize performance within these confines, relying on energy-efficient chips and streamlined algorithms to deliver supercomputer-level results from a compact package.
The Broader Implications
FSD’s advancements in edge computing ripple beyond autonomous vehicles. The innovations in low-power, high-performance hardware could enhance drones, robotics, and wearable tech. The optimization of neural networks for edge deployment could accelerate AI adoption in remote or disconnected environments, from rural healthcare to deep-space exploration. Even the cybersecurity measures developed to protect FSD systems—where a hack could be fatal—set new standards for edge device integrity.
Moreover, FSD exemplifies the synergy between edge and cloud. While the car operates independently, cloud-based simulations and fleet data continuously refine its algorithms. This hybrid model—edge for execution, cloud for evolution—could become a blueprint for future technologies, balancing autonomy with connectivity.
Challenges Ahead
Despite its promise, FSD as an edge computing marvel faces hurdles. Regulatory frameworks lag behind technological progress, and public trust hinges on proving safety beyond human drivers. Hardware must keep pace with software ambitions, and ethical dilemmas—like how a car prioritizes lives in a crash—require solutions as robust as the tech itself.
Conclusion
Full Self-Driving is more than a transportation revolution; it’s a testament to edge computing’s potential. By processing vast, complex data in real time, under stringent constraints, and with stakes as high as human lives, FSD pushes the boundaries of what’s possible at the edge. As this technology matures, it won’t just redefine how we drive—it will shape the future of decentralized intelligence across industries. In the race to autonomy, edge computing isn’t just along for the ride; it’s in the driver’s seat.